Marko Miletic
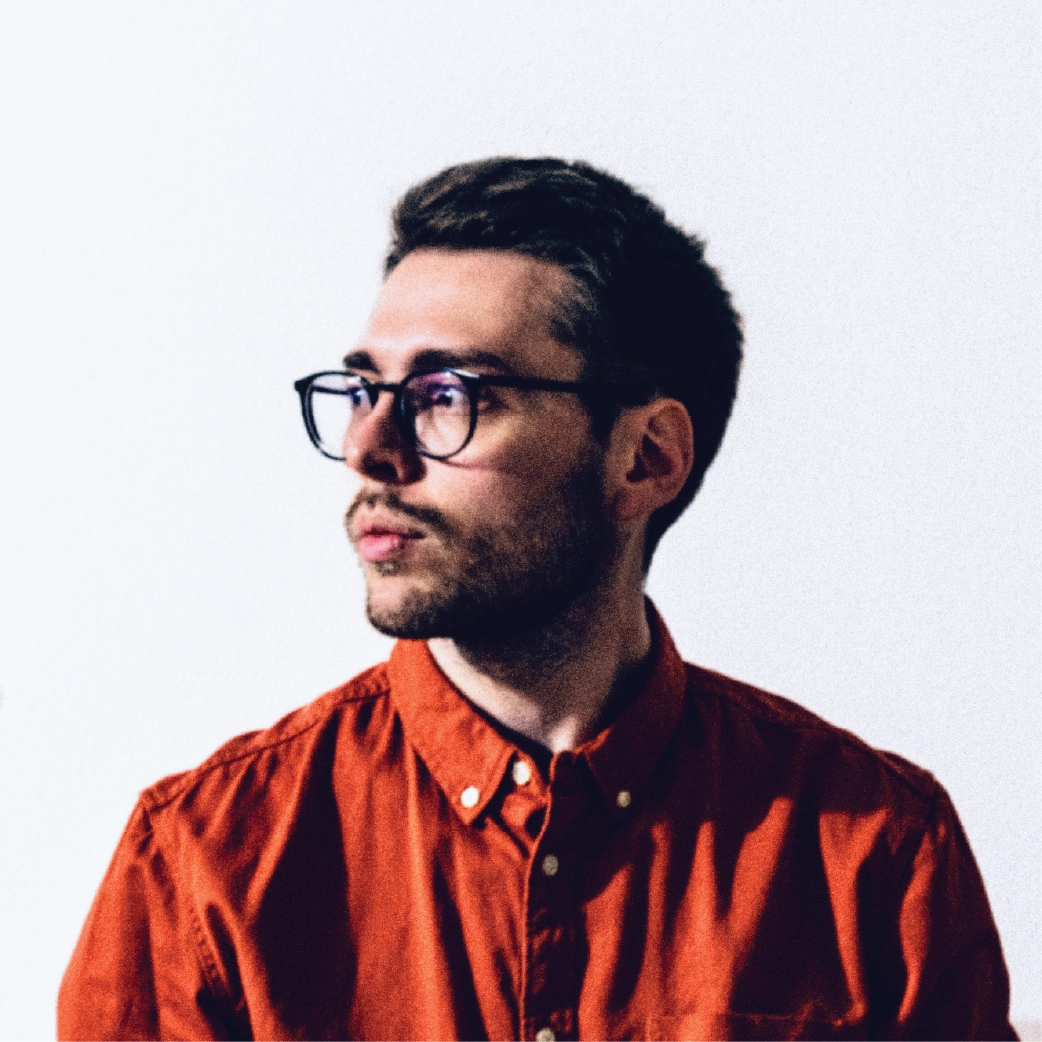
Heya! Welcome to my website.
news
latest posts
Jun 01, 2025 | Recap of The Mobi 100 Jackpot ⛰️🏃 |
---|---|
Apr 28, 2025 | The Orange and Other Small Miracles |
selected publications
- JMIR AIUtility-based Analysis of Statistical Approaches and Deep Learning Models for Synthetic Data Generation With Focus on Correlation Structures: Algorithm Development and ValidationJMIR AI, Mar 2025
Background: Recent advancements in Generative Adversarial Networks and large language models (LLMs) have significantly advanced the synthesis and augmentation of medical data. These and other deep learning–based methods offer promising potential for generating high-quality, realistic datasets crucial for improving machine learning applications in health care, particularly in contexts where data privacy and availability are limiting factors. However, challenges remain in accurately capturing the complex associations inherent in medical datasets. Objective: This study evaluates the effectiveness of various Synthetic Data Generation (SDG) methods in replicating the correlation structures inherent in real medical datasets. In addition, it examines their performance in downstream tasks using Random Forests (RFs) as the benchmark model. To provide a comprehensive analysis, alternative models such as eXtreme Gradient Boosting and Gated Additive Tree Ensembles are also considered. We compare the following SDG approaches: Synthetic Populations in R (synthpop), copula, copulagan, Conditional Tabular Generative Adversarial Network (ctgan), tabular variational autoencoder (tvae), and tabula for LLMs. Methods: We evaluated synthetic data generation methods using both real-world and simulated datasets. Simulated data consist of 10 Gaussian variables and one binary target variable with varying correlation structures, generated via Cholesky decomposition. Real-world datasets include the body performance dataset with 13,393 samples for fitness classification, the Wisconsin Breast Cancer dataset with 569 samples for tumor diagnosis, and the diabetes dataset with 768 samples for diabetes prediction. Data quality is evaluated by comparing correlation matrices, the propensity score mean-squared error (pMSE) for general utility, and F1-scores for downstream tasks as a specific utility metric, using training on synthetic data and testing on real data. Results: Our simulation study, supplemented with real-world data analyses, shows that the statistical methods copula and synthpop consistently outperform deep learning approaches across various sample sizes and correlation complexities, with synthpop being the most effective. Deep learning methods, including large LLMs, show mixed performance, particularly with smaller datasets or limited training epochs. LLMs often struggle to replicate numerical dependencies effectively. In contrast, methods like tvae with 10,000 epochs perform comparably well. On the body performance dataset, copulagan achieves the best performance in terms of pMSE. The results also highlight that model utility depends more on the relative correlations between features and the target variable than on the absolute magnitude of correlation matrix differences. Conclusions: Statistical methods, particularly synthpop, demonstrate superior robustness and utility preservation for synthetic tabular data compared with deep learning approaches. Copula methods show potential but face limitations with integer variables. Deep Learning methods underperform in this context. Overall, these findings underscore the dominance of statistical methods for synthetic data generation for tabular data, while highlighting the niche potential of deep learning approaches for highly complex datasets, provided adequate resources and tuning.